Everything you need to know about geospatial climate projections
An overview of using daily (geospatial) climate projections that are available up until 1 Jan 2100
Assessing what the climate will be like in the future is a massive challenge. We need to know how things like temperature and CO2 emissions will evolve based on different scenarios — including scenarios where we do absolutely nothing at all.
The chart below attempts to do just that.
It presents different scenarios, called ‘SSPs’ (more on these later), which demonstrate how our climate will respond to various situations up until 2100.
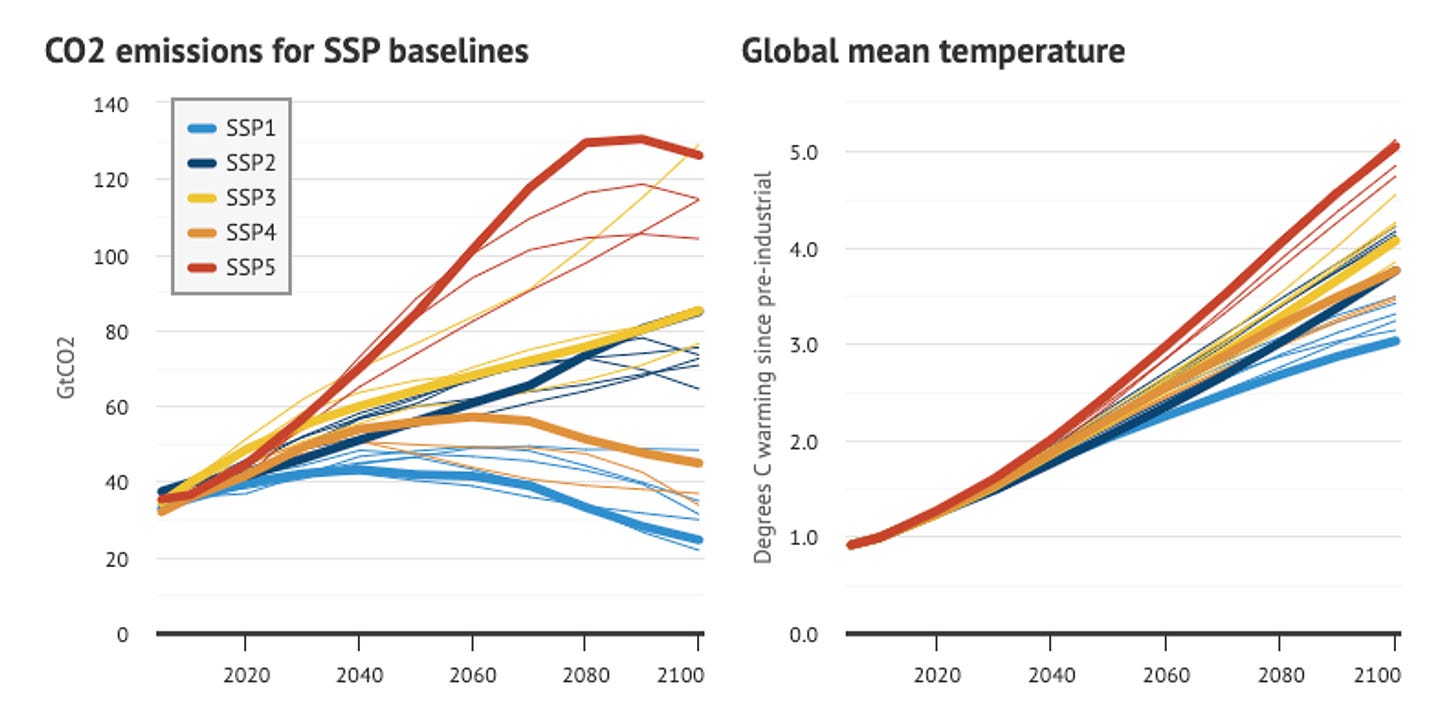
The key takeaway here is that there’s a huge gap between the red and light blue scenarios — both in terms of CO2 emissions and temperatures.
And while these graphs above show aggregated global emissions and global temperatures until 2100, we actually have this data at a more granular—i.e. geospatial—level:
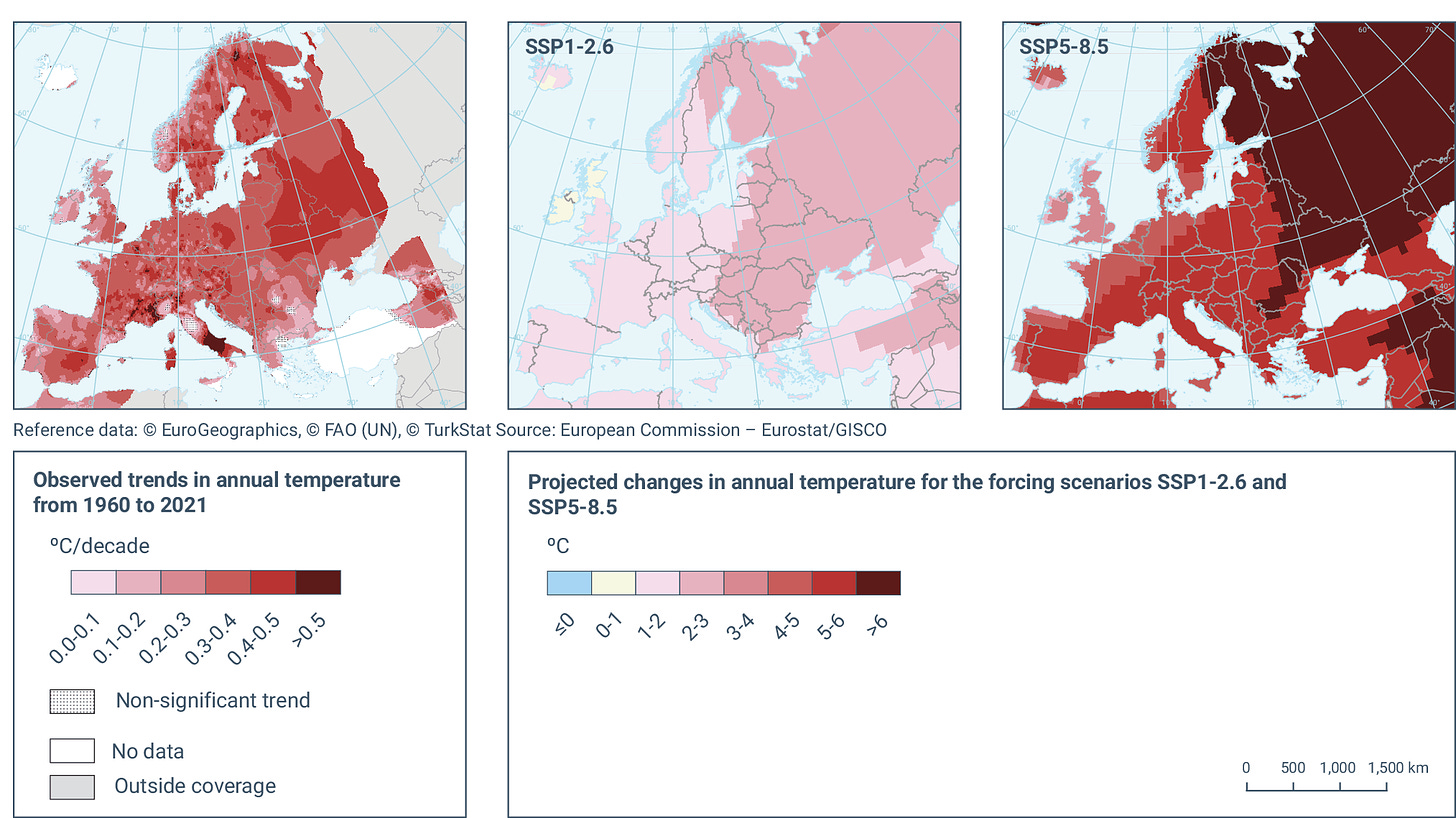
These geospatial projections are provided by an initiative called CMIP.
In fact, CMIP provides hundreds of geospatial environmental projections, for dozens of climate factors.
As a result, we can’t just use any CMIP model.
We need to understand what these models are, and how they work.
This then allows us to use the correct geospatial CMIP dataset for our purposes.
Introducing CMIP
The Coupled Model Intercomparison Project (CMIP) is an international initiative that started 20 years ago to compare different global climate models.
It’s essentially a framework for research centres to create models that simulate the atmosphere, oceans, land surface, and sea ice to understand climate behaviour.
There have been a number of ‘phases’ of CMIP, but the sixth one (CMIP6) involved 53 research centres from around the world coming together to create 134 different climate models.
Types of Data Available Through CMIP
CMIP provides a bunch of important geospatial data (historical and projections) for several climate factors. For the projections, they provide daily geospatial predictions up until 1 January 2100.
The climate models provide projections for the following variables:
Temperature
Precipitation/Snowfall
Ocean Changes (e.g. ocean temperature, acidity, and currents)
Atmospheric Composition: (e.g. concentrations of greenhouse gases and aerosols)
The full list of variables is here:
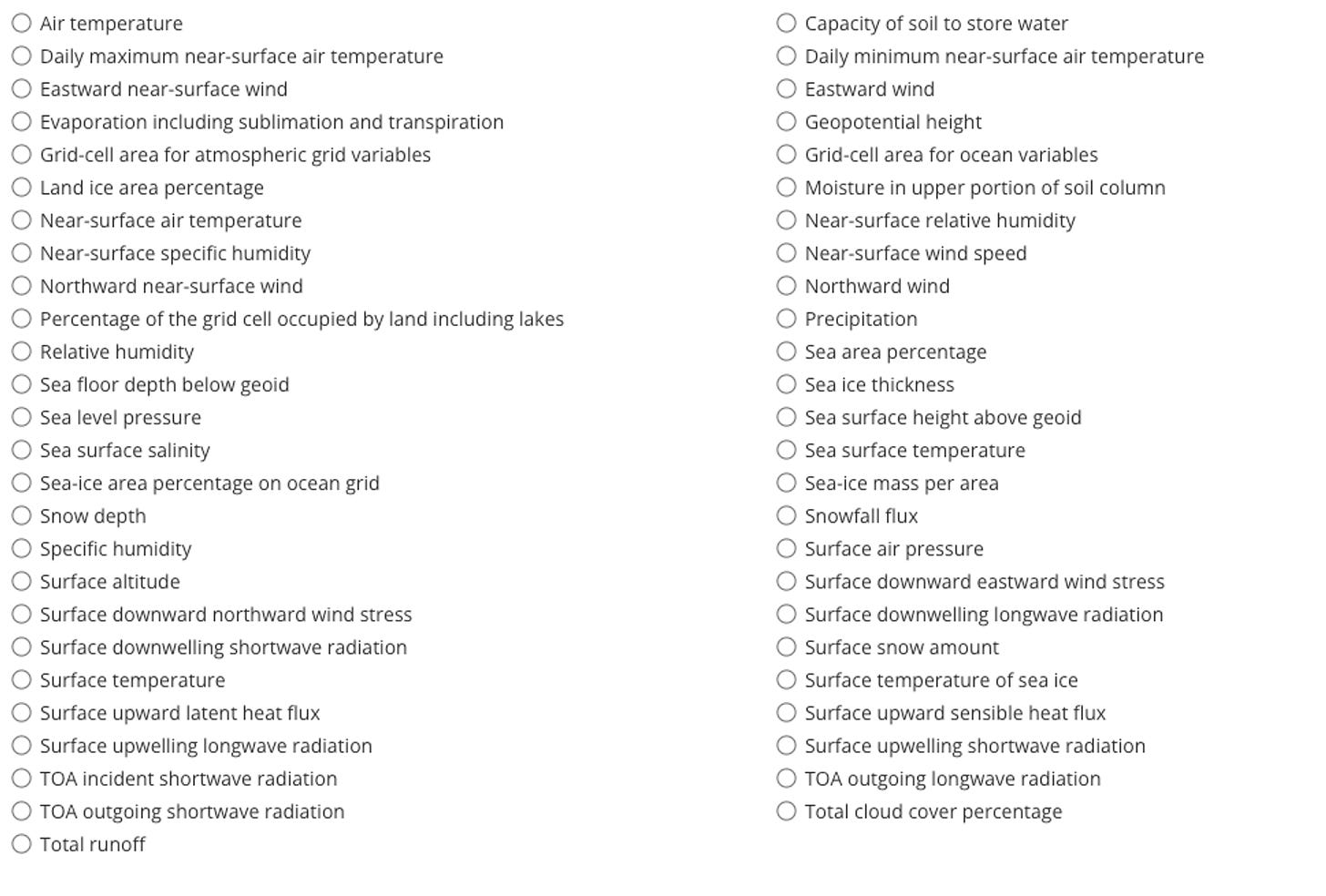
The main value adds of CMIP
You may be wondering why CMIP is so important. I.e. couldn’t any climate researcher just create their own climate models?
Well yes, but there’s a couple of important benefits of creating climate models through CMIP:
Standardisation — by standardising data outputs and model scenarios, it’s easier for other researchers to evaluate/fact-check each model’s performance, and to replicate the models (for robustness testing).
Collaboration — by setting up a framework, CMIP brings together universities and meteorological agencies across the world. This is far better than research institutes and agencies competing to create different models under different scenarios.
How CMIP’s models work
So by now you’ve got a general sense of the overall process.
To quickly recap, there are three steps we’ve seen so far:
Research centres around the world create a bunch of climate models
These models are standardised, so their outputs are the same (i.e. geospatial projections of precipitation, temperature, etc.).
All of these models use the same type of model to produce daily projections.
The models which each research centre uses are called General Circulation Models (GCMs).
What are GCMs?
GCMs simulate the physical processes of the Earth's atmosphere, ocean, and land surface. They’re pretty much the standard way of understanding how the climate system works and predicting how it’ll change over time.
There are a few different parts of GCMs:
Physical Foundations—GCMs are based on fundamental laws of physics (e.g. the conservation of energy, mass, and momentum). They integrate equations from fluid dynamics, thermodynamics, and radiative transfer to model the atmosphere, oceans, and land surface.
Differential Equations—The core of a GCM is a set of differential equations derived from these physical laws. These equations are broken down into smaller, discrete parts over a 3D grid that covers the globe. This grid is used to compute variables such as temperature, wind, humidity, and pressure at various points in the atmosphere and ocean.
Component Integration—GCMs are typically combined with models of other components of the Earth system, such as sea ice, land surface processes, vegetation, and biogeochemical cycles, to form Earth System Models (ESMs). Each component interacts within the model to provide a simulation of global dynamics.
These models are then fed with a few different inputs:
Initial Conditions—these include the current state of the atmosphere, oceans, and land surfaces. Initial conditions are usually derived from observed data (e.g. historical remote sensing data).
Boundary Conditions—these are fixed external conditions (i.e. parameters), that the model doesn’t predict but are necessary for running simulations. E.g. the composition of the atmosphere (such as CO2 concentrations), solar radiation, and geological features.
Forcings—External ‘forcings’ are drivers of climate change that are imposed on the model. These can include greenhouse gas emissions, aerosols, and land use changes. Forcings can vary over time in scenarios to study different future climate outcomes.
How ‘forcings’ are used to model the environment
Forcings are a pretty important concept when it comes to understanding how these climate models work.
In simple terms, ‘forcings’ are external factors that influence the Earth's climate system.
When you see headlines discussing how carbon emissions influence global temperatures, they’re referring to a 'forcing.' Here, CO2 acts as a 'forcing' that changes various elements of our climate, including temperatures, sea levels, and rainfall patterns.
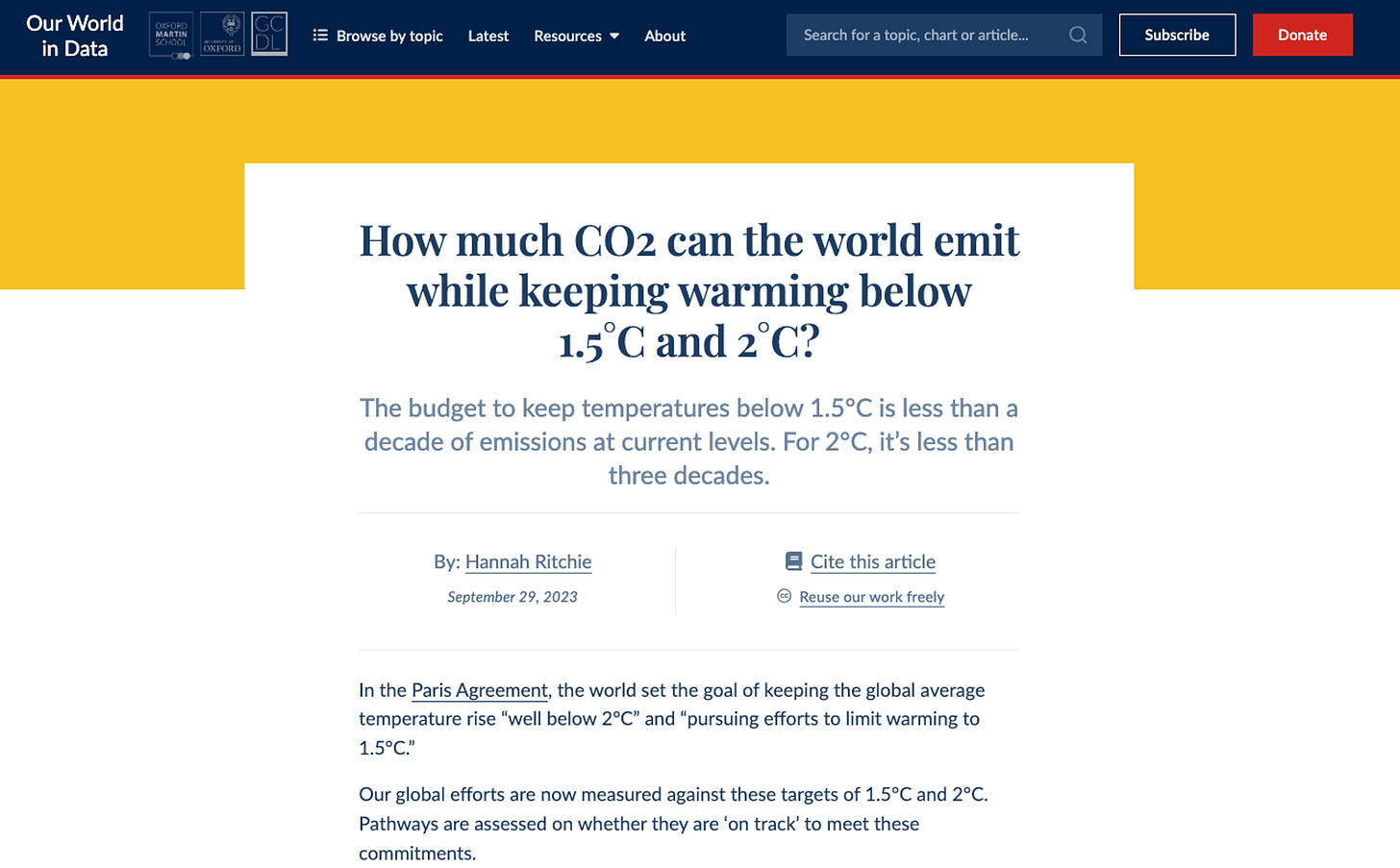
CMIP’s simulations estimate climate outcomes (like future temperatures) using scenarios that consider various factors that affect forcings like CO2 levels.
For instance, there may be simulations on how different government policies or changes in population growth could influence CO2 emissions and, consequently, global temperatures.
In fact, such scenarios are categorised under two main frameworks:
Shared Socioeconomic Pathways (SSPs) and
Representative Concentration Pathways (RCPs).
Framework 1: Shared Socioeconomic Pathways (SSPs)
SSPs are future scenarios that predict how different socioeconomic factors might change by 2100. These factors include population growth, urban development, and economic growth — all of which, in turn, influence GHG emissions.
The SSP scenarios provide five different ‘narratives’, related to socioeconomic changes and energy consumption. These can be summed up as follows:
SSP1: Sustainability – Taking the Green Road
The world gradually adopts more sustainable and inclusive ways of development
Focus on improving health, education, and the environment.
People start valuing well-being over economic growth, leading to less inequality and reduced use of materials and energy.
SSP2: Middle of the Road
Global trends in society, economy, and technology continue as they have historically.
Development and income growth are uneven across different countries.
Efforts to achieve sustainability make slow progress, environmental quality worsens in some areas, and resource use decreases slightly.
Population growth stabilizes later in the century, and income inequality remains a challenge.
SSP3: Regional Rivalry – A Rocky Road
Nationalism and regional conflicts lead countries to focus more on internal and nearby issues.
Security becomes a major focus, leading to less cooperation globally.
Investment in education and technology slows down, economic growth is sluggish, and inequality remains high or increases.
Environmental problems worsen in certain areas.
SSP4: Inequality – A Road Divided
There are growing gaps in wealth and opportunities, leading to more inequality both within and between countries.
A small part of society advances in high-tech industries, while many remain in lower-paying jobs with less education.
Environmental efforts are focused on helping wealthier areas, and technology development varies widely between sectors.
SSP5: Fossil-fuelled Development – Taking the Highway
The world relies heavily on competitive markets and technological innovation, leading to fast growth in economy and technology.
This path includes significant use of fossil fuels and high-energy lifestyles.
Despite this, local environmental issues like air pollution are well managed, and there is confidence in solving larger social and environmental problems through technology.
As a result, when we examine the projections, we see that the SSP5 scenario (Fossil-fueled Development) is projected to have almost three times the CO2 concentration by 2100 compared to the SSP1 scenario (Taking the Green Road).
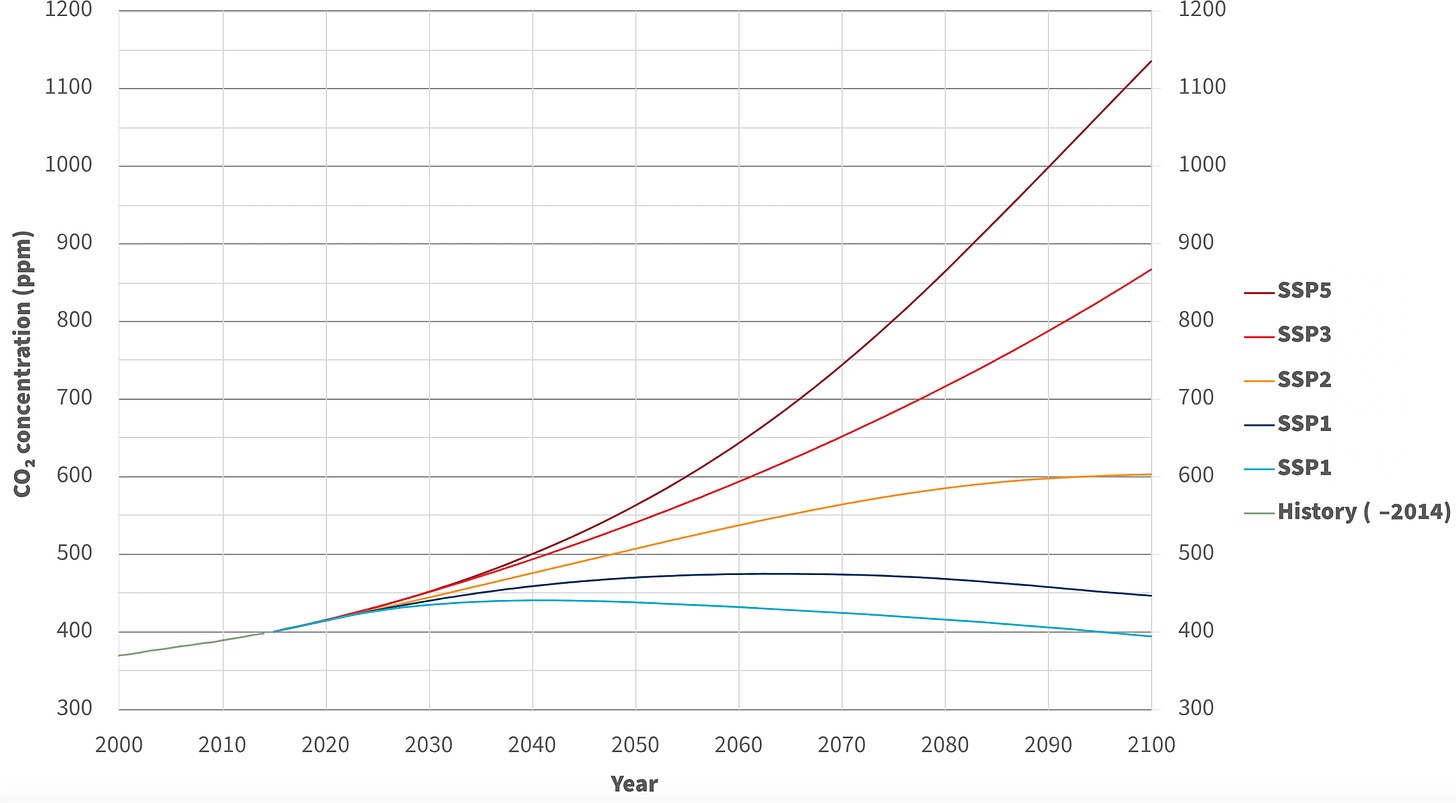
In fact, the Sixth Assessment Report of the UN’s Intergovernmental Panel on Climate Change (IPCC), came up with estimates of global temperature estimates until 2100 for these SSP scenarios.
Given the Paris Agreement's goal to limit global warming to below 2°C above pre-industrial levels, it seems that only the SSP1 scenario, which involves reducing emissions to net zero by 2050, is likely to get us there.
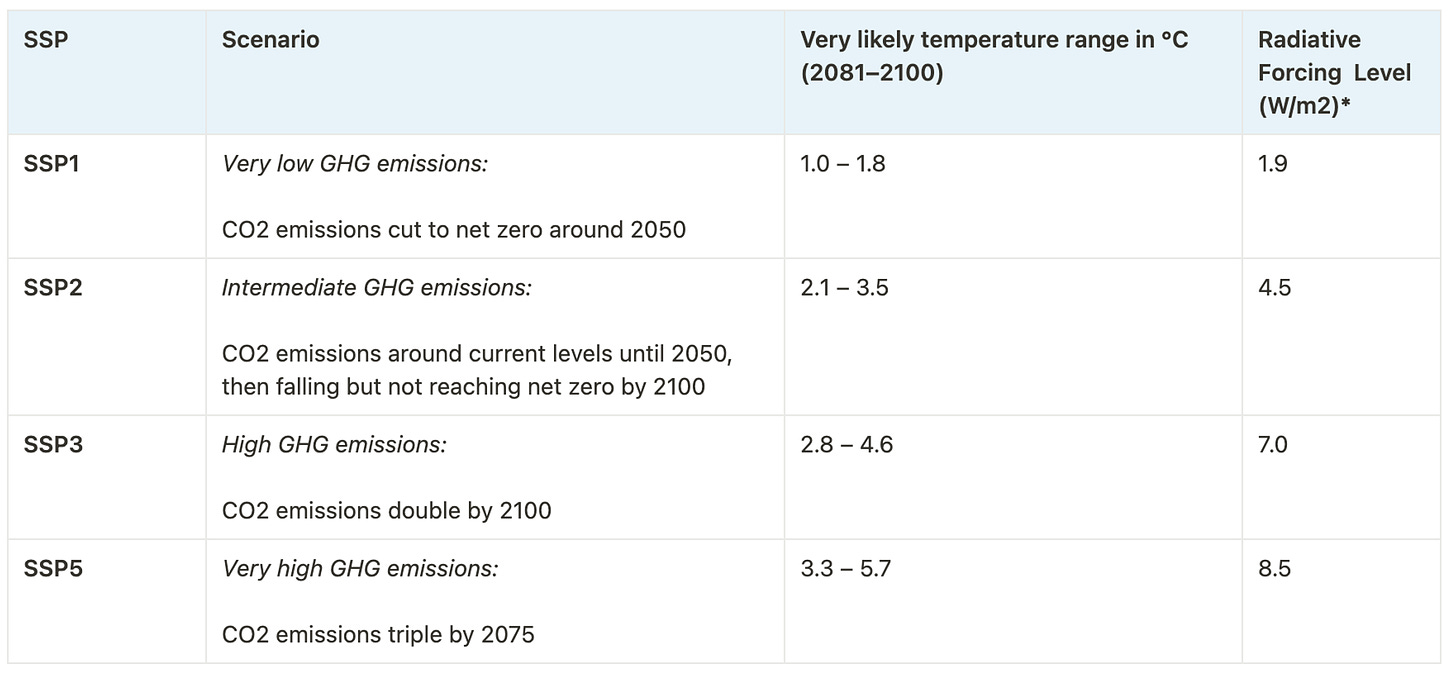
Framework 2: Representative Concentration Pathways
Whereas the SSPs combined socioeconomic ‘narratives’ with forcings; the Representative Concentration Pathways (RCPs) focus purely on describing different levels of GHGs and ‘radiative forcings’.
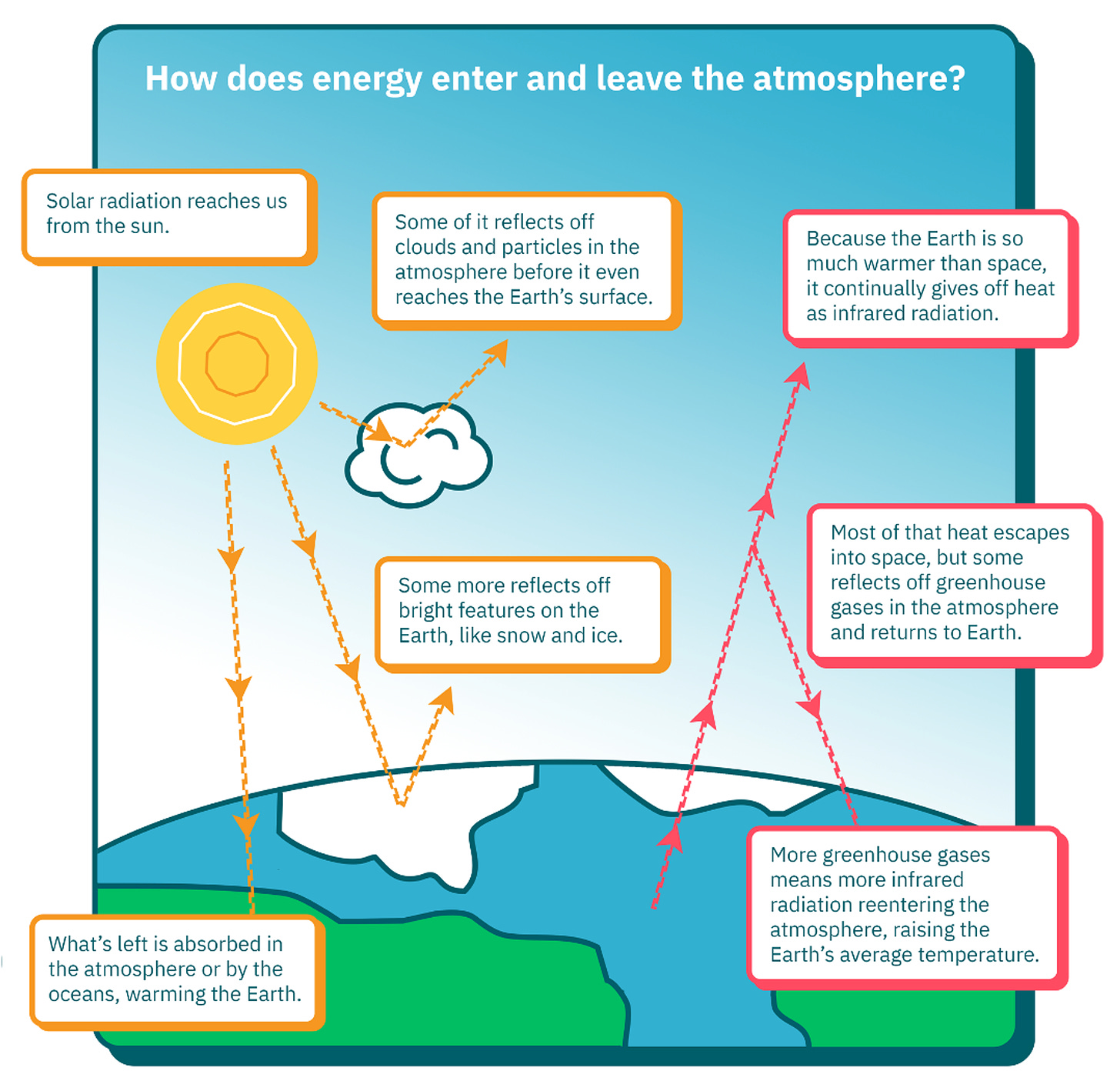
Radiative forcings are essentially a way to compare the amount of energy coming to Earth from the sun to the amount of energy that's going back out into space from Earth.
It looks at factors like greenhouse gases that can change this balance. If more energy is coming in than going out, it results in warming, and if less energy comes in, it leads to cooling.
Nonetheless, given that the RCP scenarios only look at GHGs and other radiative forcings, the RCPs are narrower than the SSPs. This is because they don’t account for socioeconomic changes.
Nonetheless, there are a few different RCP scenarios that we need to be aware of:
RCP 1.9:
Aims to keep global warming below 1.5°C compared to pre-industrial levels.
Requires significant reductions in greenhouse gas emissions and negative emissions technologies (like capturing carbon from the atmosphere).
RCP 2.6 — a very stringent pathway:
Aims to limit warming to well below 2°C.
Involves early and rapid reductions in emissions and achieving net-zero CO2 emissions by the latter half of the 21st century.
RCP 4.5 — an intermediate scenario:
Global emissions peak around 2040 and then decline.
This pathway could result in a temperature increase of between 2°C and 3°C by 2100.
Requires substantial emissions reductions but is less strict than RCP 2.6.
RCP 6.0:
Emissions peak later, around 2080, before declining.
This scenario leads to a stabilisation of radiative forcing by 2100, with a moderate level of climate change mitigation.
RCP8.5 — rising emissions:
no significant efforts are made to curb emissions, leading to continuous increases throughout the 21st century.
Results in the highest level of radiative forcing and severe impacts from climate change.
The mean temperature projections under the RCP scenarios according to one research paper (Pielke Jr, et al., 2022) as follows:
In short, the lower RCP values are the best scenarios for the planet, but would require the strictest mitigation strategies being introduced.
How to deal with different climate models
As mentioned earlier, CMIP6 involved 53 research centres from around the world coming together to create 134 different climate models.
The annoying thing for us — as data users — is that all 134 models provide different results for their (SSP and RCP) projections of temperature, precipitation, etc.
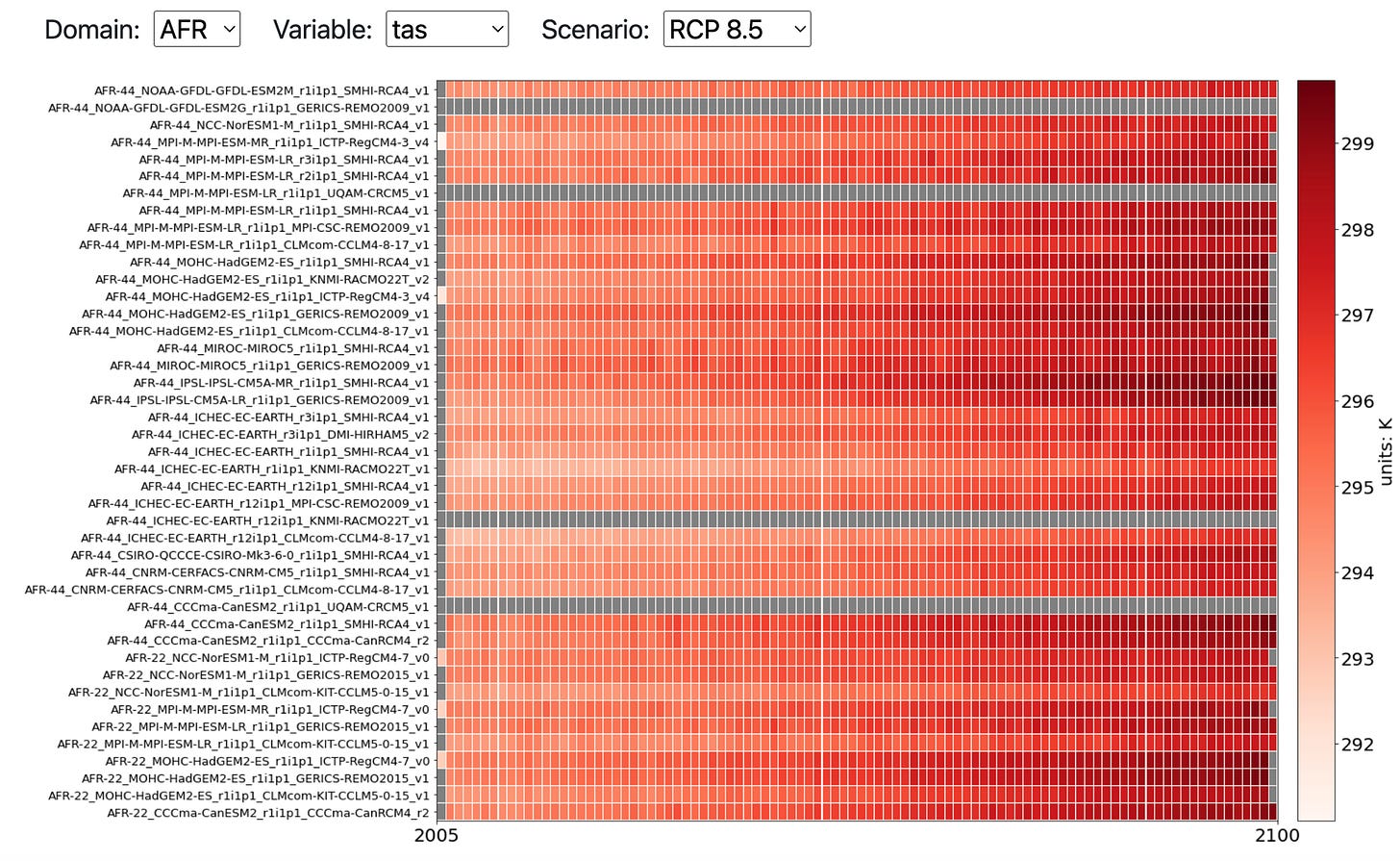
This (slightly confusing) infographic shows how different models predict global average temperatures under the RCP 8.5 scenario.
Each row in the image represents a different model. Darker red indicates higher temperatures. The vertical axis starts in the year 2005 and ends in 2100. By 2100, you can see variations in temperature predictions, with some models showing much lighter reds than others.
This gives you a sense of how different these projections can be.
Ensembling as a solution
Given these differences in climate models, it’s natural to wonder which model we should be using.
There are two approaches to this:
Understand the assumptions, strengths and weaknesses of all 134 models
Ensemble the models together
For many of us who are looking to get a general sense of climate projections, ensembling the models together (e.g. taking the average) is probably the simplest approach. In fact, it’s what the World Bank recommends as part of its Climate Change Knowledge Portal.
This also saves us from the painstaking task of going through all of the individual assumptions for each model.
How to access the data
Now that we understand how to work with this data, all that’s left is to access it.
You can download the geospatial datasets from the Climate Data Store at this link.
Conclusion
To sum up, in this article we’ve covered:
What type of geospatial climate projections are available — i.e. temperature, wind, precipitation, etc.
How this data is created — through General Circulation Models
What the various climate scenarios are — i.e. Shared Socioeconomic Pathways and Representative Concentration Pathways
How to deal with multiple models — the simplest solution is through multi-model ensembling (e.g. taking an average across all the models).
How to access the data — through the Copernicus Data Store
With this information, you’ll be in a good position to use geospatial projections on a range of climate factors for your work and research.
Hi, Yohan.
Thanks for your posts and newsletter, it´s how I keep updated on geospatial topics. Great content!
Regarding the RCPs, I am not sure they "don’t account for socioeconomic changes". When they released them, they didn't publish the socio-economic parameters that accompany the RCPs, but they are accounted for when producing the RCPs. There is a way we reach different future emission levels, after all. The SSPs, as I understand it, are the successors of the RCPs rather than a complement. More info in section 3.1 in this paper: https://doi.org/10.1007/s10584-011-0148-z . Let me know what you think.
Cheers,
Ignacio