Rapidly assess damage to buildings in conflict zones
PLUS: Using satellites to measure Tesla's oversupply of cars, assessing food security in Ukraine, and new super-resolution datasets.
Hey guys, here’s this week’s edition of Spatial Edge — a weekly round-up of geospatial news that’s completely free. Think of this as more like the newsletter equivalent of QGIS than ArcGIS… The goal is to help make you a better geospatial data scientist in less than 5 minutes.
In today’s newsletter:
Damage Detection: A new tool identifies war-damaged buildings using SAR data.
Ukraine Food Security: NASA maps artillery craters affecting cropland.
Sen2NAIP Dataset: High-res and low-res image pairs for super-resolution training.
GEE Community Catalog: New datasets for agriculture, palm oil, fires, and rivers.
Tesla’s Oversupply: Satellite images reveal Tesla's car inventory buildup.
Research you should know about
1. Rapid Damage Mapping
Let’s face it. There’s a lot of conflict happening around the world at the moment. And with all this conflict, humanitarian organisations need to know where to provide assistance.
A new tool (and paper), from researchers at ETH Zurich, University of Zurich and the ICRC, uses remote sensing and machine learning to detect where war-damaged buildings are located. It uses SAR data (from Sentinel-1) and open building footprints to create damage estimates for each building in an area.
If you’re interested in a different approach to assessing damage using Sentinel-1 (and InSAR), check out this paper. In simple terms, it measures changes in how similar radar signals are from one day to another (this is known as ‘coherence’). If the radar signals are massively different (i.e. they have ‘low coherence’), then it’s likely that a building has been damaged by war. The codebase is available here.
2. Measuring food security in Ukraine
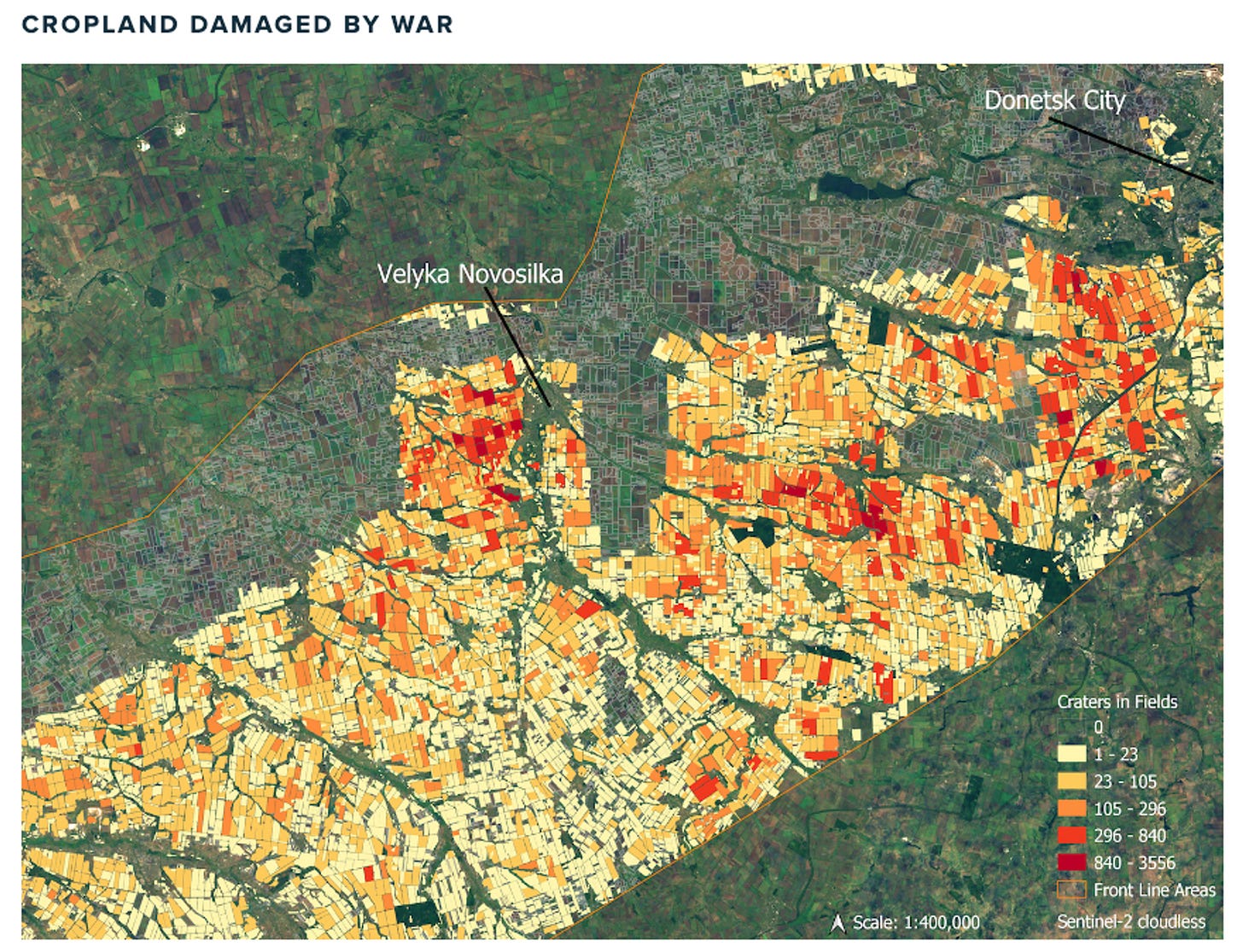
NASA Harvest has used remote sensing data to assess the impact of the Ukraine war on its food security.
Specifically, they found:
Russia occupied about 22% of Ukraine’s cropland.
Despite predictions, 90% of the wheat crop was harvested in 2022, and most cropland was planted with spring crops.
About 7.5% of Ukraine’s cropland was abandoned in 2023, which could have fed 25 million people for a year if planted
3. Querying catalogues of satellite data using images AND text
If you’ve got a huge catalogue of satellite data, it can be a nightmare to find stuff you’re interested in. It’s essentially a needle in a haystack.
A new paper has introduced a way to search through satellite images using both image examples and text descriptions. So, if you have an example image of a plane and type in the word “purple”, you’ll be returned with satellite images that contain purple planes in them.
This is one way of tackling the ‘search’ problem in remote sensing. In a similar way to how Google ‘structured’ the internet by making it searchable, many researchers are aiming to do something similar with satellite images.
Geospatial Datasets
1. A new dataset to train super-resolution models
ESA’s OpenSR team (from Oxford and the University of Valencia), have created a new dataset, Sen2NAIP.
They made the dataset by first finding 2,851 pairs of high-resolution images from the National Agriculture Imagery Program (NAIP) and low-resolution images from Sentinel-2. These image pairs were selected to be cloud-free and taken on the same date.
Then, they trained a model to transform high-resolution NAIP images to look like the low-resolution Sentinel-2 images.
Using this model, they created 17,657 pairs of high-resolution NAIP images and their transformed low-resolution versions that resemble Sentinel-2 images (S2like).
The takeaway
This method allows us to massively increase the number of high-resolution and low-resolution image pairs, which can be used to train super-resolution models.
2. M3LEO dataset that integrates InSAR and RGB data
M3LEO is a new dataset that includes diverse InSAR data from Sentinel-1 and RGB imagery from Sentinel-2. It covers 17.5TB and includes about 10 million data samples from six different regions.
3. New datasets from the GEE Community Catalog
The GEE Community Catalog has introduced a bunch of new datasets that can be used on Google Earth Engine. This includes data on:
Field Boundaries of Agriculture (for the UK)
Global Palm Oil Plantation Extent
ESA Fire Disturbance Climate Change Initiative dataset (which produces maps of global burned areas)
Global River Obstruction Dataset (maps human-made structures that obstruct river flow)
4. SARDet-100k
SARDet-100K is a large-scale multi-class SAR object detection dataset. It includes 116,598 images and 245,653 instances spread across six distinct categories: Aircraft, Ship, Car, Bridge, Tank, and Harbor.
Other interesting bits
Using satellite data to measure Tesla’s oversupply of cars: This super-interesting article measures the slowdown in demand for Teslas from satellites. They do this by measuring the number of ‘surplus’ cars that are parked at the Gigafactory in Austin. They compared images from October 2022 (top image) and May 2024 (bottom image).
A new report from Deloitte and the World Economic Forum on Earth Observation claims that the economic opportunity from Earth Observation insights will surpass $700 billion by 2030. EO data will also supposedly contribute to the abatement of 2 gigatonnes of greenhouse gases annually.
A Comprehensive Benchmark for Optical Remote Sensing Image Super-Resolution. There’s a lot of fluff in the super-resolution space. One way to address this is to ensure there are standardised methods of assessing the quality of super-resolution models. That’s what this paper (from ESA’s OpenSR team) proposes.
OpenEO now supports the creation of Sentinel-2 Level-2H: As mentioned in the last edition of the newsletter, ESA’s Sen2Like model allows us to create a harmonized Sentinel-2 and Landsat-8/9 time series. We can now use this model on OpenEO’s cloud computing resources.
Geospatial Jobs
The United Nations Department of Economic and Social Affairs is looking for two geospatial information offices to be a part of the Secretariat for the Committee of Experts on Global Geospatial Information Management. You can check out the opportunities here and here.
The University of Edinburgh is looking for a couple of lecturers in Physical Geography.
That’s it for this week.
I’m always keen to hear from you, so please let me know if you have:
new geospatial datasets
newly published papers
geospatial job opportunities
and I’ll do my best to showcase them here.