Some surprising facts about nightlights data
Nightlights are often used but not always understood. Let's change that here.
One of the most common questions I’m asked is whether I can recommend any good overviews of nightlights data.
I don’t know of anything, so I’ve decided to put one together.
Many of us have heard that nightlights can be used as a proxy for economic activity. The idea is that higher levels of luminosity correlate with more economic activity in a place.
For example in this image we can see how bright places like Paris, Madrid and Barcelona are compared to the rest of France and Spain:
However, some of the details about nightlights are less well-known.
For example:
What do we mean by economic activity?
What type of economic activity is captured by nightlights?
Where is economic activity actually generated?
Is the relationship between luminosity and economic activity linear?
Can it accurately capture economic activity in rural areas?
What are some of the confounding factors when using nightlights?
So in this newsletter, I’ll be addressing these questions.
Onwards.
Using nightlights to measure economic activity
There were a few studies in the 1990s and 2000s that looked at using nighttime luminosity as a proxy for economic activity such as Elvidge et al. (1997), and Doll et al. (2006).
However, a paper published in the prestigious economics journal, the American Economic Review (Henderson et al., 2012), marked an inflection point.
It argued that growth rates of nightlights were useful in two scenarios:
When countries lacked high-quality official GDP estimates;
Where official sub-national GDP was unavailable
Additionally, they argued that luminosity data is available immediately, as opposed to official GDP data which can have delayed publication of a few months.
This study led to a bit of a frenzy in the economics space. And now nightlights has become the default method of measuring sub-national economic activity.
Now that nightlights are so frequently used, many studies don’t even go into the details of what it captures, and what its strengths and limitations are.
So in light of this, let’s take a look at some of the key questions.
What economic activities do nightlights capture?
It’s quite vague to say that nightlights captures ‘economic activity’. What exactly do we mean by economic activity?
In the Henderson et al. paper, they use nightlights as a proxy for real GDP growth.
But taking a step back, GDP is typically measured in three ways:
Adding up all of the consumption in an economy (this includes investments and government spending)
Adding up all of the income earned in an economy
Adding up the value of all things produced in an economy
On aggregate for an entire country, all of these things should equal one another.
However, we need to be careful about double counting. For example, combining production values with income and consumption figures without accounting for overlaps could distort things.
Henderson et al., essentially view nightlights as a measure of nighttime consumption:
“Intensity of night lights reflects outdoor and some indoor use of lights. However, more generally, consumption of nearly all goods in the evening requires lights. As income rises, so does light usage per person, in both consumption activities and many investment activities.” — Henderson et al. (2012).
But the reality is a bit more complex. Nightlights can also be used to capture some production-related activities such as nighttime construction and nighttime mining.
When we look at nightlights for the Pilbara mining region in Australia, we not only see lights generated from mines being lit up at night (i.e. production-based economic activity), but we also see lights generated by mining staff who are consuming stuff at night (e.g. eating out).
The bottom line: nightlights can capture certain elements of consumption and production.
However, we need to ensure that these production and consumption activities aren’t double-counting things. We also need to have an idea of how representative nighttime consumption and production are for the region overall.
For example, do people mainly consume stuff during the day? Or is it an equal split between night and day?
Answering such questions is pretty important for accurately using nightlights to measure ‘economic activity’.
Spatial mismatches between production and consumption at night
Imagine I work in London but live in Essex, an hour away. My work (i.e. production) contributes to London's economy, but when I spend time in Essex, like eating out at night, that's where my spending (i.e. consumption) mainly happens.
In this example, my economic activity (i.e. my job) in London doesn't get picked up by nightlights. However, the places where I spend money at night in Essex, like restaurants, do light up and are visible from space.
This essentially creates a discrepancy between areas where economic activity is generated during the day (London) versus at night (Essex). This isn’t a big deal if we’re using nightlights to measure economic activity in large regions (e.g. the whole of England). This is because I’m most likely generating economic activity during the day in England and spending money at night in England.
However, with nightlights we can zoom into areas as small as 500m2. As a result, it's pretty likely that I work during the day in one 500m2 area and spend money in a different 500m2 area at night. Therefore, this implies that pixels with higher daytime economic activity will be systematically underrepresented in nightlights data. This underrepresentation occurs even if these areas generate a lot more economic activity, such as financial districts, compared to areas bustling with bars and restaurants, which tend to be overestimated in nightlights data.
The point here is that the more you zoom in, the bigger these spatial mismatches between daytime and nighttime economic activity become.
This reflects a concept called the ‘Modifiable Areal Unit Problem’ (MAUP). This happens when the results are impacted by how we group data.
There are two main types of MAUP:
The scale effect: This is about how data changes when it’s grouped at different levels of aggregation. For example, in the image below, grouping data by small districts might show that 21% of census districts have a high density of children. But if we look at counties, only 9% do.
It's the same data, but the story is slightly different.
The zonal effect: This is where the results are impacted by how you draw boundaries. In this example, electoral boundaries can be re-drawn to favour the blue party instead of the red party.
For example here, in each grouping, the blue colour has more dots per group.
However here, in three of the groupings, the red colour has more dots.
The bottom line: the level of granularity for nightlights analysis really matters.
The ratchet effect
The "ratchet effect" may occur when lights, once installed, remain on or aren't removed, even in economic downturns. So while nightlights can effectively capture economic activity in booms, they may become less reliable during recessions, as illumination persists despite economic downturns.
This is something that Henderson et al. look into.
They find virtually identical coefficients for both positive and negative deviations in light intensity. Or to put it simply, they find that changes in luminosity (whether increases or decreases) are equally predictive of changes in economic activity.
Hence they rule out the existence of a ratchet effect.
This is something that I’ve noticed too - particularly during the pandemic.
I created this graph of luminosity for cities in Europe, and we clearly see luminosity responding to events like localised lockdowns.
This is a no-brainer really—previously bustling cities had become virtual ghost towns during the pandemic. I remember walking through Covent Garden in 2020, amazed to experience the place with only about 10 other people who ventured out to grab a socially distanced takeaway burger from Five Guys.
Non-linearities of nightlights
While nightlights can reflect economic changes during growth and recessions, it raises another question: Is the relationship between nightlights and GDP linear?
In other words, does a one-unit increase in GDP translate to a proportional increase in nightlights?
The short answer is no.
The more complex answer requires a bit more information about the nightlights data used.
As discussed by Bickenbach et al. (2016), Henderson et al. use the DMSP-OLS nightlights dataset. This is a low-resolution dataset that spans from 1992-2014. It has a resolution of 1km. This dataset rates luminosity on a scale from 0 (no light) to 63 (very bright), with the highest values capped at 63.
This top coding has a specific implication. For example, a brightly lit area, such as in New York, might be recorded at the maximum value of 63 in 1992. If its actual brightness doubled by 2000, this increase wouldn’t be reflected in the dataset. Instead, it would still be recorded as 63 in 2000. Therefore, beyond a certain luminosity level, the dataset fails to capture increases in economic activity through higher luminosity levels.
In addition, the dataset’s low resolution means that areas showing no light might still have some economic activities. It’s simply that these activities don’t generate enough light to be detected by the satellite.
The bottom line: due to issues with top coding and sensor sensitivity, there isn’t a linear relationship between luminosity and GDP.
Distinguishing urban vs rural areas
Given these issues of detecting low levels of light, it’s probably no surprise that these DMSP-OLS images struggle to accurately capture nightlights in rural areas.
According to a World Bank report:
DMSP lights data poorly measure rural areas. For example, Nordhaus and Chen gridded the globe into one-degree cells and found one-third of cells with positive population and output were recorded as having zero light in DMSP data.
A study was subsequently launched to understand why satellites often can’t detect lights in rural areas. They used seven very bright, 1000-watt lamps (i.e. those used in large warehouses) in rural Colorado and New Mexico. The lamps were enhanced with aluminium shields to point the light toward the sky.
However, despite these measures, the DMSP satellites could only detect the lights about half the time. This is a problem given that the luminosity from these lamps was significantly more than the brightness of typical village lighting.
The bottom line: the low-resolution DMSP satellite images struggle to capture luminosity in rural areas.
Other sources of noise in nightlights data
However, while I’ve discussed issues with the low-resolution (DMSP) satellite images, the higher-resolution (VIIRS) data is much more accurate.
It doesn’t have top-coded luminosity values, and it’s much more sensitive to luminosity in rural areas.
I’ve previously done a pretty comprehensive overview of VIIRS data — you can read about it here.
However, in a nutshell, VIIRS nightlights data is double the resolution (~500m) of the DMSP-OLS data (~1km).
We can see the improvement in resolution when comparing these two images of Delhi in 2012:
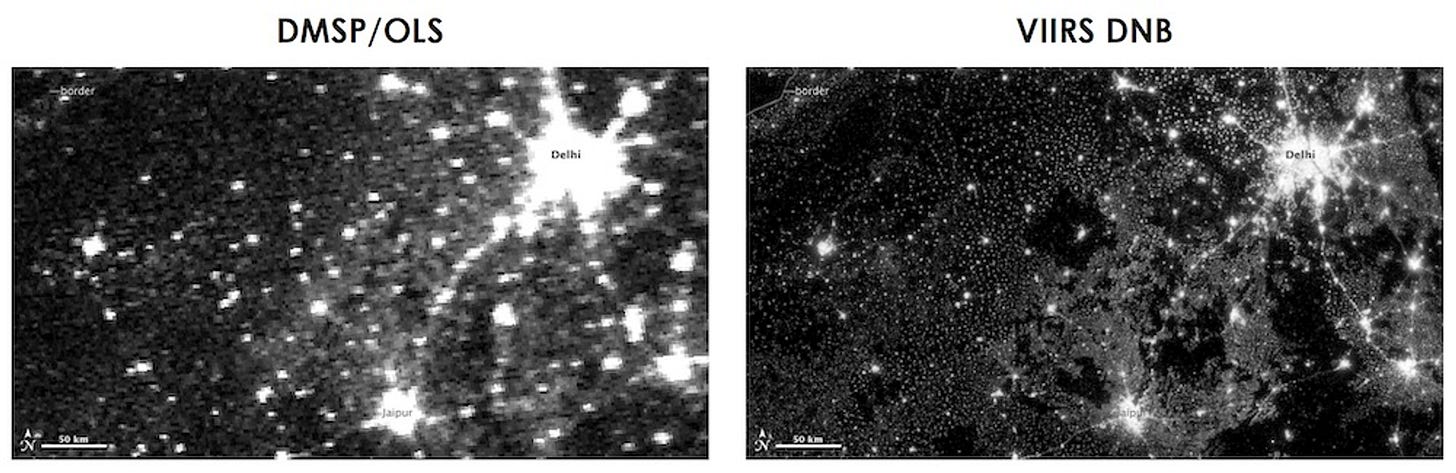
Nonetheless, these high-resolution images also experience sources of noise that can confound its luminosity scores.
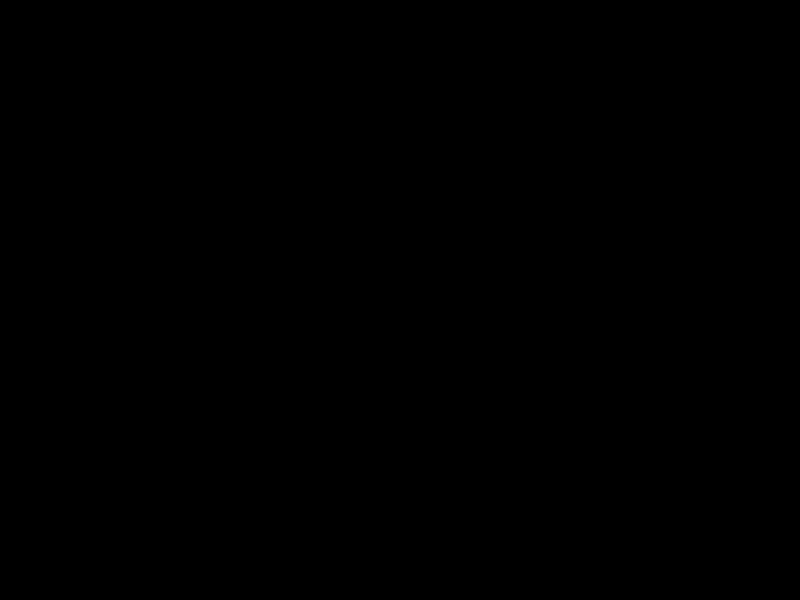
As I described in my previous article, these sources of noise include:
cloud cover
light reflecting off snow
the angle of the satellite
light reflecting of aerosols in the atmosphere
stray light (which comes from the sun directly into the satellite’s instruments)
These are all factors that need to be addressed when using nightlights. If you’re wondering how to do this, I actually provide a step-by-step tutorial on how to control for these factors using NASA’s nightlights images here.
The bottom line: high-resolution (VIIRS) satellite data doesn’t suffer from the same issues as the lower-resolution data (i.e. top-coding and an inability to detect rural luminosity). However, it needs to be appropriately cleaned and processed to address other sources of noise.
How does LED lighting impact nightlights data?
LEDs are a more energy-efficient source of lighting than the High Pressure Sodium (HPS) lights that are typically used in outdoor lighting.
However, LEDs have a shorter wavelength. In the graph below, we see that LEDs (blue line) have shorter wavelengths than HPS lights (yellow line):
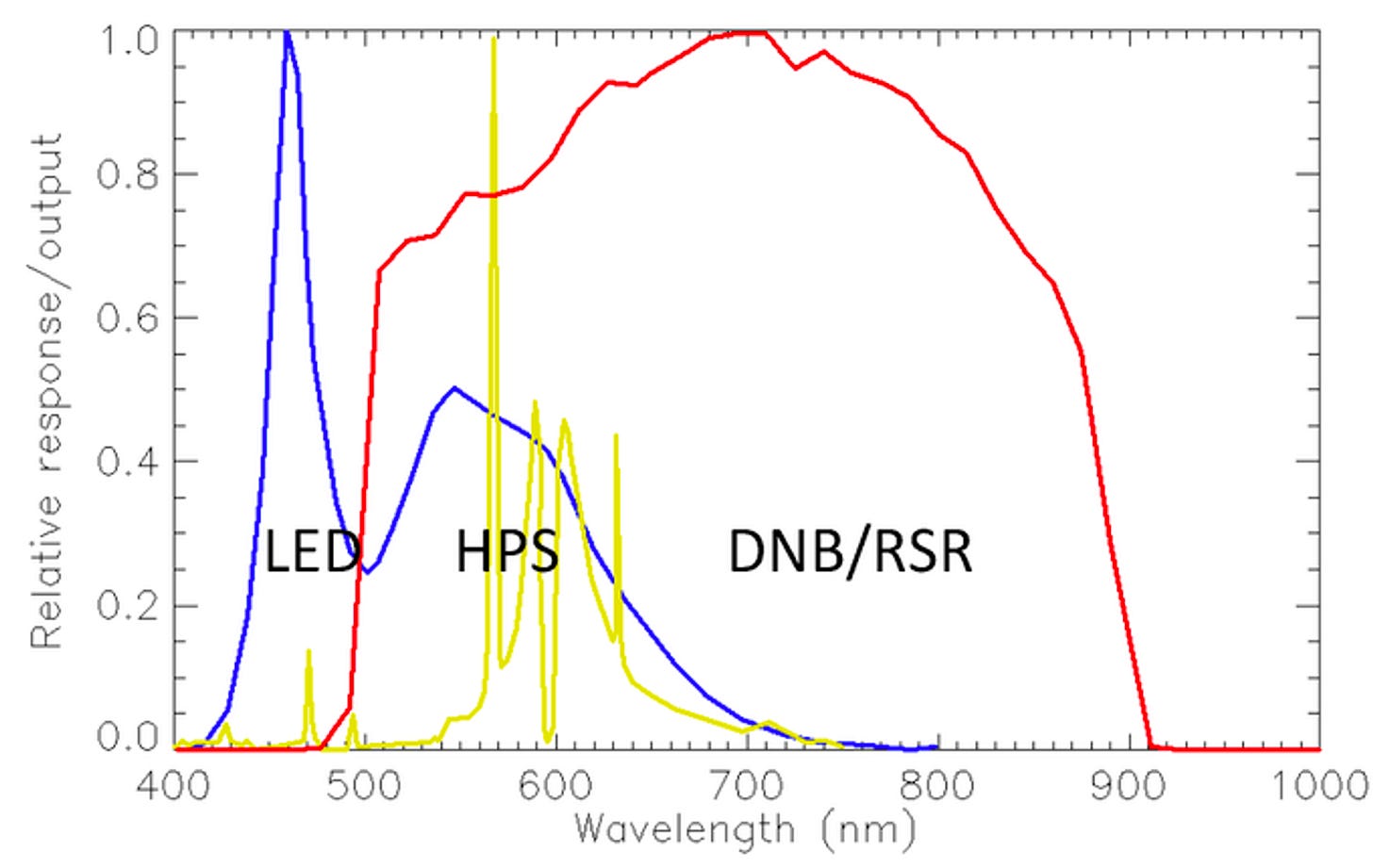
However, VIIRS satellites can’t capture ‘blue light’ emissions from LEDs.
In the graph below:
the blue rectangle represents the blue light spectrum
LEDs (blue line in the top panel) emit a lot of blue light
VIIRS data (blue line in the bottom panel) can’t capture any of this
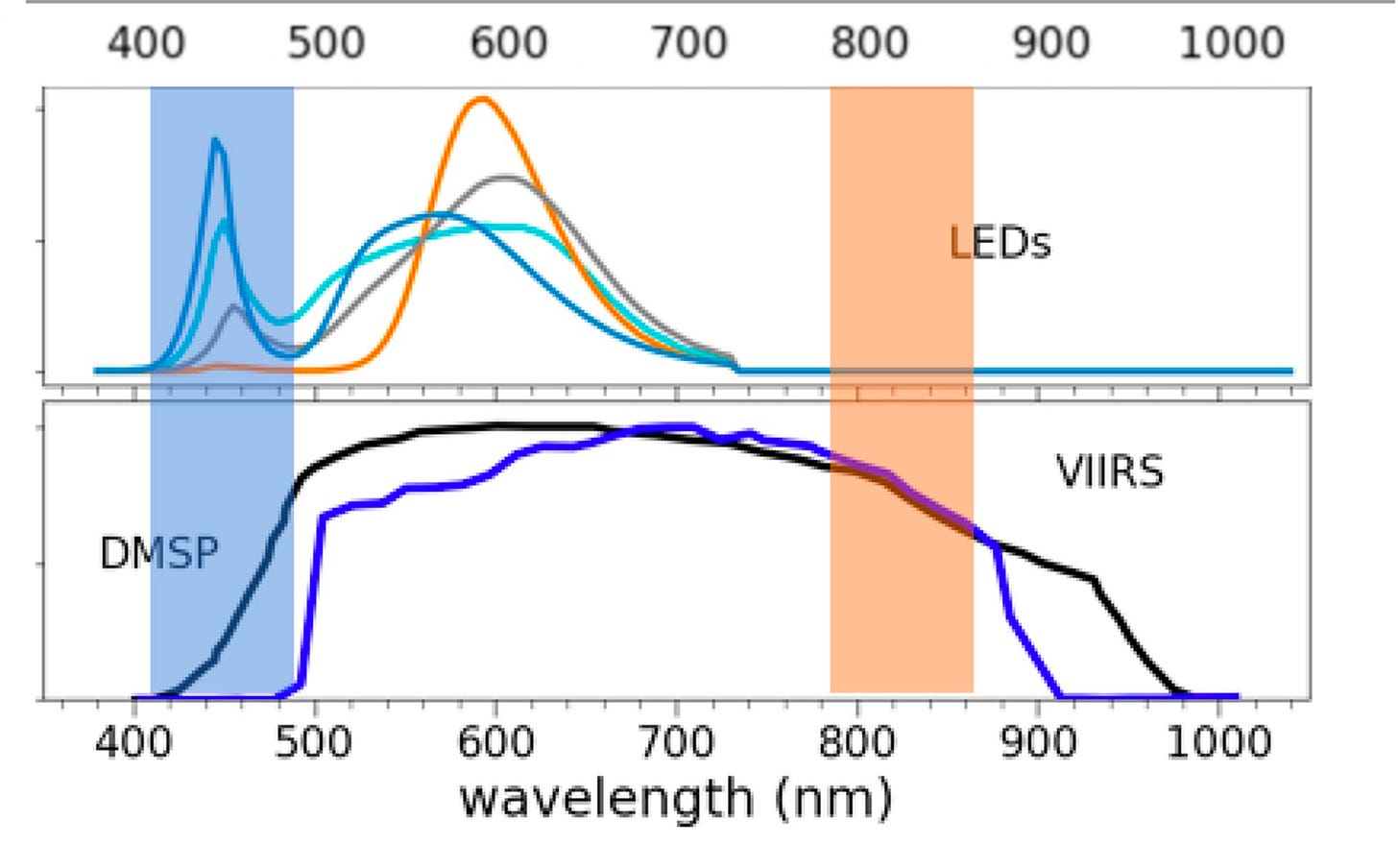
This means that as cities introduce policies to change outdoor lighting to energy-efficient LEDs, VIIRS satellites will mistake this for a reduction in luminosity.
We can see this exact thing when we look at Milan, which introduced LED lighting in 2015.
When we aggregate all luminous pixels in Milan in 2013 and 2016, we can see that luminosity appears to decrease:
However, if we look at official real GDP for Milan from Eurostat, we see that GDP actually increased from 2013 to 2016:
This is an obvious problem. In the future, we’ll be seeing more cities introducing LED lighting. VIIRS nightlights won’t be able to capture this properly, so they’ll inaccurately state that luminosity has decreased.
The bottom line: using nightlights as a proxy for GDP will become increasingly challenging as more cities introduce LED lighting.
Conclusion
My hope is that this little overview of nightlights has provided you with some more nuanced information on how we can use it to measure aspects of our economies, and what its limitations are.
I still believe nightlights are the best proxy for economic activity at a granular level. However, I do think this data can be made even better if it’s (1) cleaned and processed properly, and (2) enriched with other datasets (e.g. agricultural GDP estimates, traffic, or infrastructure data).
Sponsor
This newsletter is sponsored by Ekko Graphics, a UK-based design studio that builds websites and geospatial platforms. I’ve worked with them previously at the World Bank, and they’ve built websites and done brand design for two of my former companies, 505 Economics and Lanterne. They’re offering a free consulting call if you’re looking to set up a personal website or develop a geospatial platform.
Great piece, thanks Yohann. So have you heard about planned initiatives to supplement the below 450nm spectrum for nighttime observations?